Artificial Intelligence (AI) is ambitious and immensely beneficial for the advancement of humankind. In a space like healthcare, especially, artificial intelligence is bringing about remarkable changes in the ways we approach the diagnosis of diseases, their treatments, patient care, and patient monitoring. Not to forget the research and development involved in the development of new drugs, newer ways to discover concerns and underlying conditions, and more.
However, this is not without its fair share of bottlenecks. For an AI model to be accurate and serve its purpose, it has to consistently get trained. For this, it needs tons of AI training data and this is where problems actually start.
There isn’t much training data available as such. From the outlook, it might appear that there are mammoth volumes of data available in the form of MRI and CT scans, reports, EHRs, X-Rays, clinical trials, and a host of other unstructured data sources, the required number of datasets to train AI models always falls short.
An AI model only gets better with training and in a sector like healthcare, where precision is the only factor that stands between the life and death of an individual, rigorous AI training is the only way to roll out reliable AI models and systems.
This is exactly where synthetic data comes in. What is it? Well, we’ll explore this in detail in today’s post.
What Is Synthetic Data?
As the name suggests, synthetic refers to synthesized – something which is not naturally occurring. Synthetic data is data that is generated by computers. This is not available in surveys, forms, reports, or datasets from computer vision but is completely auto-generated.
However, it is important to understand that these synthetic datasets stem from real-world datasets and are based on their observations and inferences.
These artificially synthesized data have the following characteristics:
- They are annotated by default by machines
- They are ultra-realistic, where it is extremely difficult to differentiate them from real datasets
- And are generated in massive volumes
The onset of synthetic data is probably one of the coolest aspects of the AI revolution in healthcare.
The prominence of synthetic data is fast growing in the healthcare spectrum. Even healthcare experts and industry veterans and leaders estimate that in the next three or five years, robotic surgery will become mainstream thanks to the precision AI robots will have developed due to synthetic data. Furthermore, within a decade, such advanced robots will be deployed in mainstream healthcare centers and hospitals to perform autonomous surgeries.
For all this to happen, CXOs should make a note of synthetic data today. The seeds for tomorrow’s advancements have to be sowed today and that’s why they should work on budgeting and channelizing revenues to develop synthetic data sources for their products, devices, or models.
Use Cases and Benefits of Synthetic Data
Apart from solving the demand-supply gap in the availability of quality datasets, synthetic data solves real-world concerns in fascinating ways. Here’s a quick list to give you a quick idea of some of its use cases and benefits.
- One of the primary benefits is it enables researchers to face no hiccups in proceeding with their observations and research stemming from lack of data. They could work on their hypothesis and theories with synthetic data and use that to simulate real-world data and zero in on results and observations. From oncology to neurological studies, the research could be fast-tracked.
- The second most important benefit or use case is associated with data privacy and compliance. As you know, there are several stringent HIPAA regulatory protocols and compliances healthcare organizations need to follow and adhere to with respect to the data they generate and use. Factors like data de-identification, confidentiality, and privacy come into play. However, all these could be avoided with synthetic data seamlessly.
- Extending the previous point, replacing real-world datasets with their synthetic counterparts will also eliminate security concerns associated with their breaches or compromise. Statistics reveal that close to 40mn healthcare records have been exposed without authorization between 2020 and 2021 alone.
- Patient monitoring devices and wearable health devices could be optimized for precise prediction and prescription of concerns thanks to a secondary layer of analysis with the help of synthetic data.
- Medical imaging will get a boost with this, where injuries and instances of fire burns or minute tumors could be developed artificially in imaging reports for teaching and learning purposes in colleges and universities.
- Robotic surgery would significantly advance as robots will have super-realistic simulation scenarios in hand to perform surgeries, come up with AI-powered recommendations, and more.
- Rare diseases which are still not studied or predicted due to lack of required datasets could be studied in detail, resulting in a more accurate prediction of viral or contagious outbreaks.
Wrapping Up
We know this sounds great and quite honestly, too good to be true as well. Like any evolving entity, synthetic data faces some challenges that need to be resolved. For starters, let’s understand that synthetic data is reliant on real-world data. This means the quality of the mimicked dataset is directly proportional to its source, which also means that any inherent bias would be present in synthetic data as well.
Also, this is a new and upcoming concept. So, a lot of industry insiders wouldn’t still be open to the option of training their models with synthetic data and would rather wait till they get hands-on with real-world data. Lastly, generating synthetic data also involves time, effort and money.
It would be interesting to see what breakthroughs in this space could happen, taking the reach of synthetic data and the understanding of it to the masses.
What do you think?
Author Bio
Vatsal Ghiya is a serial entrepreneur with more than 20 years of experience in healthcare AI software and services. He is the CEO and co-founder of Shaip, which enables the on-demand scaling of our platform, processes, and people for companies with the most demanding machine learning and artificial intelligence initiatives.
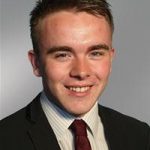
Erik Jackson has been a senior editor at Health News Tribune for three years. Fluent in French and proficient in Spanish and Arabic, he focuses on diseases and conditions and the newest trends in medicine.